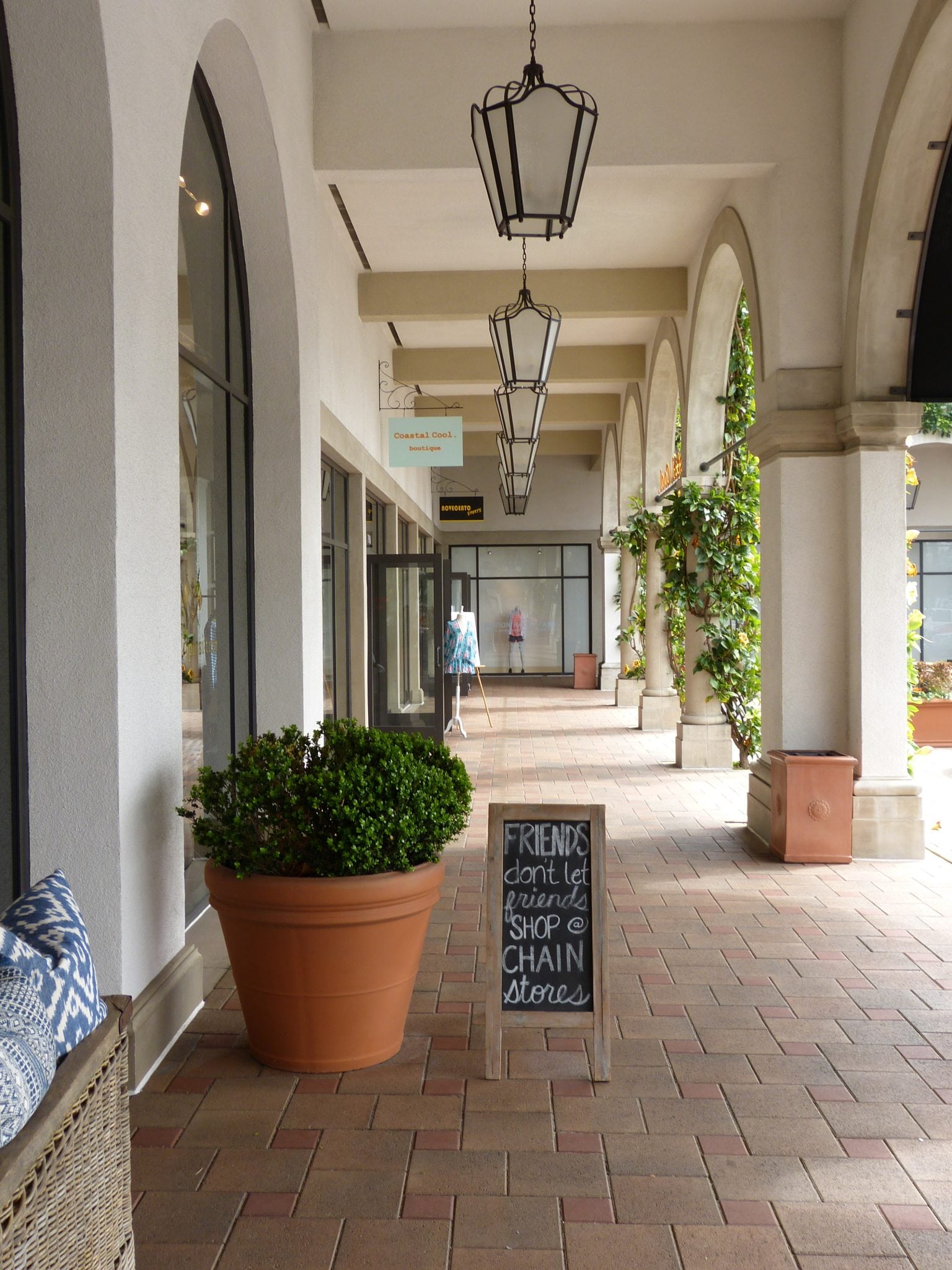
Retail’s Transformation: From Business Intelligence to Data Science
Jonathan Beckhardt, co-founder and GM of insights, DataScience
When was the last time you met someone without a smartphone, laptop or other digitally-connected device? When was the last time you went somewhere without using your own devices for an extended period? These technologies are now almost inescapable, creating mountains of valuable consumer data that brands are taking advantage of to better understand customers’ behaviors and spending habits.
By analyzing consumer big data, retailers are able to pinpoint trends and translate them into business practices to increase engagement and profits. While the practice is paying off for many brands, making the leap from business intelligence to data science can be confusing and intimidating. However, avoiding this change ensures that retailers miss out on revenue opportunities.
Take the leap with confidence by avoiding these top five mistakes.
Ignoring predictive modeling
Before predictive analytics technology, retailers often had to rely on sales records and other historical data to find trends in consumer behavior and spending, then make educated guesses as to how they should apply them to future practices. However, customers today are making decisions based on a variety of complex factors, including personalized recommendations and digital promotions, making insights difficult to identify. That’s where predictive modeling comes in. Using predictive models, retailers can evaluate how all of these different customer data points relate to each other, and how they individually — or collectively — impact their key metrics. For instance, an online retailer can build a model that evaluates the totality of a customer’s behavior, from email opens to the sequence of items added to a cart, to infer how much money the customer is likely to spend over the course of their lifetime. From this, a retailer can determine how many marketing dollars should be attributed to that customer segment.
Hiring the wrong talent for critical roles
Hiring the wrong talent is a costly mistake for any organization, but it can be particularly damaging for retailers, who often have to rely on a single expert to fill many of the company’s data engineering and analysis roles. Those roles tend to fall into two categories: data engineers and data scientists. Data engineers are responsible for building solutions for working with data, while data scientists derive value from that data to meet broader corporate objectives and identify trends. However, data scientists hold a very specialized skill set, one that makes them adept at compiling and analyzing information, and that knowledge isn’t required for the work done by most engineers. While the two work intimately with one another, their knowledge and skill sets don’t overlap, making it difficult for one person to fill both roles. Instead, by making the investment to hire the necessary number of data specialists, retailers are better able to make data-driven decisions and positively impact their business.
Focusing on a limited data set
With the mountains of consumer data available today, companies can easily become overwhelmed or side-tracked by the sheer size of the information presented to them. In many cases, this can lead to focusing on an overly narrow or limited data group in order to simplify the data collection and analysis process. Applying the results of this analysis to the wider customer base or company policy, however, can create a skewed image and even negatively impact the retailer’s operations. Instead, by strategically combining the results of analyses on a number of categories, such as event-stream, user and feedback data, retailers are able to uncover more accurate and effective strategies for attracting, engaging and retaining their customers. Before jumping into big data analysis or even data science, it is important that these companies determine the necessary size and scope of their analytics program in order to yield the most accurate and useful insights to meet their business’ objectives.
Putting profits ahead of customer experience
Omnichannel customers, or those who shop across a variety of channels both online and in-store, have been shown to spend 15 to 30 percent more than other consumers. These shoppers also exhibit stronger feelings of loyalty toward brands, even recruiting others to shop with that retailer. As the number and availability of digital channels continues to grow (think: you can buy pizza by texting an emoji now), failing to track customers’ interactions across each of the touchpoints means that valuable options to increase engagement can go completely unnoticed. Alternatively, by collecting purchase and customer feedback across the spectrum of digital channels, companies can better understand how shoppers prefer to interact with the brand, as well as which avenues deliver both the most positive sentiment and highest ROI. It is true that profit will always remain the priority for retailers, but a customer’s experience is what ultimately drives him or her down the path to conversion.
Failing to invest in the right data architecture
If the case hasn’t been made already, investing in the right data architecture tools is becomingly increasingly essential to retailers’ success in the era of big data. Without a centralized data warehouse and the right tools and expert employees to collect, analyze and interpret data, retailers will only have access to a small piece of the valuable information it contains. Ultimately, gaining access to truly valuable information is contingent upon a company’s ability to refine and comb through the initial data coming in. Making that kind of investment up front can mean putting immediate profit on hold, a difficult prospect for smaller retailers — but the value will reveal itself in both long term ROI and customer longevity.
By 2018, Gartner predicts that more than half of all large organizations will be using advanced analytics to compete in the marketplace. The trend toward data science will only continue to accelerate, making it all the more important that retailers take the first step today to integrate the right infrastructure, analytical tools, and data specialists into their standard business practices.
By Jonathan Beckhardt, DataScience. As Co-Founder and General Manager of Insights, Jon oversees the data science and insights teams at DataScience. He previously served as product manager at Tuition.io, where he developed payment optimization algorithms and data visualization tools to simplify student loans. Jon is a graduate of Oberlin College (B.A) and the University of Southern California (M.B.A.).
Clark Bateman
Som great in depth insights here. I feel like conversion optimization is often overlooked, especially at the small business level. We use yellowpagesdigital.com for our digital marketing needs, and it wasn’t until we started seeing all of the new traffic that we weren’t taking advantage of that we started to understand the need for CRO and being able to madify key selling points by looking at the data and making informed decisions. We were just simply acting in ignorance beforehand.